Surrender in Data Science and AI: Embracing Change for Innovation
- Mozhgan Tavakolifard
- Nov 28, 2023
- 7 min read
Updated: Nov 29, 2023

In the realms of data science and artificial intelligence (AI), 'surrender' emerges as a paradoxical yet profoundly significant concept. Far from indicating defeat, surrender here symbolizes a metaphorical gateway to innovation, adaptability, and unprecedented growth. It represents the readiness to abandon outdated methodologies in favor of novel ideas, adapting to the ever-changing technological landscape. I was invited as a speaker and panelist for WiDS conference (Women in Data Science) in October where I talked about "Surrender and AI: Embracing Change for Innovation".
In this post, I explore how surrender, viewed as openness to change and adaptability, is pivotal in navigating the complexities of AI and data science and for innovation. We will examine the historical progression of AI, emphasizing transformative shifts necessitated by the surrender of previous limitations. We'll delve into how surrendering in data science means transcending traditional statistical models, adopting machine learning algorithms, and allowing unbiased data to guide decision-making. As we embark on this exploration, we unravel the essence of surrender as a strength, a catalyst for continuous learning, ethical practice, and ultimately, mastery in AI and data science.
The Evolution of AI: A Journey Through Paradigm Shifts
Early Enthusiasm and the First AI Winter
The advent of AI was greeted with a surge of optimism in the 1950s and 1960s, driven by the belief that replicating human intelligence was imminent. Significant developments, like the creation of the first neural networks and the Turing test, laid the groundwork for AI's potential.
However, this initial enthusiasm met the harsh realities of technological limitations, leading to the first AI winter in the late 1970s. The dominant reliance on rule-based expert systems, effective in structured tasks, failed in complex real-world problems. The field's response was a paradigm shift - a surrender of old methods for new possibilities.
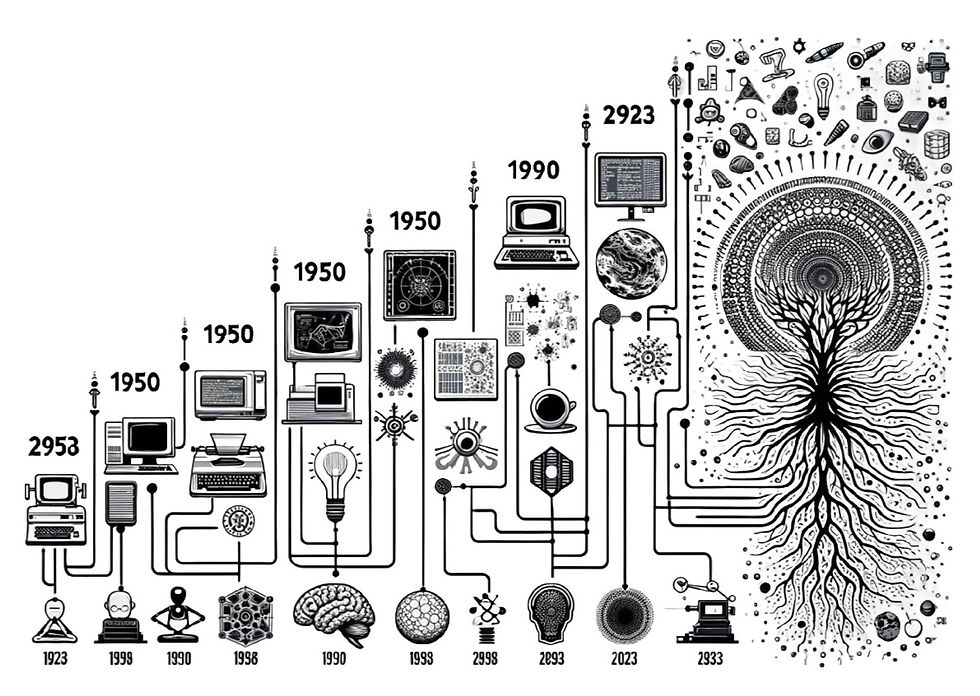
The Rise of Machine Learning and the Second Wave
The resurgence of AI interest in the late 1980s and 1990s marked a significant shift from rule-based systems to machine learning. This period introduced AI systems capable of learning from data and making decisions autonomously, with breakthroughs like backpropagation in neural networks enabling previously unattainable applications.
Yet this era also faced setbacks, leading to a second AI winter in the early 2000s. Even sophisticated machine learning models had limitations, highlighting the need for continual adaptation and surrender to evolving methodologies.
Deep Learning and the Current Landscape
Today's optimism in AI is fueled by deep learning advancements. Deep learning models have excelled in tasks like image and speech recognition, often surpassing human performance and powering innovations like autonomous vehicles and sophisticated language models.
Despite these advances, the field faces ethical, privacy, and environmental challenges. The future of AI hinges on our ability to surrender outdated paradigms in favor of sustainable, ethical approaches.
Surrendering in Data Science and AI, Accepting Complexity and Uncertainty, Embracing Change for Innovation
Data science has evolved from relying on rigid statistical models to adopting machine learning algorithms capable of processing vast amounts of data and uncovering complex patterns. This transition is not just about methods but a fundamental shift in mindset, accepting the complexity of the world and finding answers within that complexity.

Data science now prioritizes data-driven decision-making over traditional hypothesis-driven approaches. This shift demands the surrender of preconceived notions, allowing surprising, data-led findings to guide hypotheses and conclusions. This approach values empirical evidence over established doctrine, promoting objectivity and accountability in insights derived from data. Read also my other post on Redefining Transformation in Business and Self, AI-driven Transformation.
The Flexible and Adaptable Nature of AI
AI's evolution from rigid, rule-based systems to flexible, data-driven learning models represents a significant surrender. Early AI attempted to replicate intelligence through exhaustive rules, but the complexity of the real world necessitated a new approach - machine learning from data and experience, adapting to unpredictable scenarios.
This surrender led to AI systems that could learn and adapt autonomously, aligning more closely with natural intelligence. The shift was not just technological but philosophical, accepting uncertainty and complexity as inherent in intelligence.
Continual Learning: The Heartbeat of Modern AI
AI's rapid advancements necessitate a philosophy of continuous learning and evolution. Staying at the forefront of innovation means constantly updating one's knowledge and skills, surrendering outdated practices for new developments.
This journey extends beyond technical knowledge, encompassing the ethical, societal, and philosophical implications of AI. As AI becomes increasingly integrated into our lives, professionals must engage with complex questions surrounding privacy, bias, autonomy, and the nature of intelligence.
Business Strategy for Data-Driven Transformation
Regarding business strategy, the idea of surrendering to data-driven insights implies a dynamic approach to decision-making. Companies that are able to pivot based on what the data tells them can maintain a competitive edge. Netflix is a prime example, having transitioned from a DVD rental service to a streaming giant and content creator based on user data and viewing habits. Another example is Spotify.
In my own consulting experience, the contrast between companies that rigidly stick to their plans versus those that adapt based on data is stark. The latter tend to be more successful because they respond to actual market conditions and customer behaviors rather than assumptions and predictions. By constantly analyzing data and making informed decisions, these adaptable companies can quickly identify trends, spot opportunities, and address challenges in real-time.
In contrast, companies that rigidly stick to their plans often find themselves struggling to keep up with the ever-changing business landscape. They may be too focused on following their pre-determined strategies, even if those strategies are no longer effective or relevant. This inflexibility can result in missed opportunities, wasted resources, and ultimately, a decline in competitiveness.
Adaptable companies, on the other hand, thrive by embracing a data-driven approach. They gather and analyze various types of data, including market research, customer feedback, and sales metrics, to gain insights into what works and what doesn't. This allows them to make informed decisions and adjust their strategies accordingly.
Moreover, adaptive companies understand that consumer behaviors and market conditions are dynamic. They proactively monitor and respond to these changes, ensuring that their products, services, and marketing efforts align with current demands. This flexibility enables them to stay ahead of the curve and maintain a competitive edge.
Another advantage of adaptability is the ability to seize new opportunities as they arise. By closely monitoring market trends and customer preferences, adaptable companies can identify emerging needs and develop innovative solutions to meet them. This agility allows them to capture new market segments, expand their customer base, and drive growth.
The contrast between companies that rigidly stick to their plans and those that adapt based on data is significant in consulting. Adaptable companies, by responding to actual market conditions and customer behaviors, tend to be more successful. Their data-driven approach enables them to make informed decisions, quickly adapt to changing circumstances, and seize opportunities for growth. Read also my other post on Seven Pillars of Data-driven Business Growth.
Challenging Established Norms in AI Projects: Embracing Innovation
Innovation in AI is often sparked by challenging the status quo or what we term "Brules" (bullshit rules, this term is from the book "The Code of Extraordinary Minds" by Vishen Lakhiani). This process is not just about adopting new technologies but also about fostering a mindset that embraces questioning and critical thinking.
Questioning Assumptions and Norms
The heart of innovation in AI lies in questioning the underlying assumptions and biases within projects. Are there implicit beliefs or standard practices that limit thinking? By examining these assumptions, AI practitioners can identify areas ripe for innovation and improvement.
Encouraging Diverse Perspectives
Diversity in AI teams is not just about varying technical skills but also about incorporating a range of experiences and viewpoints. This diversity can lead to challenging established norms and fostering creative problem-solving. Teams should be encouraged to include members from different backgrounds and disciplines, as this can bring fresh perspectives to AI projects.
Experimentation and Adaptation
Innovation in AI also requires a willingness to experiment with new algorithms, data sources, or methodologies. This experimentation might lead to failures, but these are often stepping stones to breakthroughs. An environment where experimentation is encouraged and where failure is seen as a learning opportunity can significantly drive innovation forward.
Ethical Considerations: The Cornerstone of Responsible AI
As AI becomes more integrated into various aspects of life, ethical considerations become increasingly important. Balancing technological innovation with ethical responsibility is essential for sustainable development in AI.
Prioritizing Fairness and Bias Mitigation
AI systems can unintentionally perpetuate or amplify biases present in training data. It is crucial to prioritize fairness and actively work to identify and mitigate biases. This process involves using diverse datasets and constantly evaluating AI systems for biased outcomes.
Ensuring Privacy and Transparency
AI systems often deal with sensitive personal data. Upholding privacy and ensuring data security is paramount. Transparency in AI processes also builds trust. Users should have access to information about how AI systems make decisions, especially in critical applications like healthcare or criminal justice.
Accountability in AI Development
AI developers and companies must be accountable for their systems' impacts. This responsibility includes addressing any harm caused by AI systems and being responsive to public concerns. Developing ethical guidelines and adhering to them is crucial for maintaining public trust in AI technologies.
Shu-Ha-Ri Framework: Mastering AI and Data Analytics
The Shu-Ha-Ri framework is a concept originating from Japanese martial arts, representing the stages of learning and mastery. It's often used metaphorically to describe the journey of skill acquisition in various disciplines:
Shu (Obey): In this initial stage, the learner strictly follows and replicates the teachings and techniques of their mentors without deviation, focusing on foundational skills and knowledge.
Ha (Detach): The learner begins to diverge from traditional teachings, experimenting and adapting what they've learned to develop their own style and interpretations.
Ri (Transcend): In the final stage, the learner transcends existing frameworks and rules, achieving mastery and contributing new insights and innovations to the field.
In my approach, I've adapted the traditional Shu-Ha-Ri framework to the context of business transformation, emphasizing its relevance in today's data-driven landscape.
Shu: Embracing Foundational Practices
In the Shu phase, businesses focus on understanding and integrating foundational data science and AI practices. This stage is about building a solid base and leveraging proven methods and technologies to establish a strong footing in the digital arena.
Ha: Experimenting and Adapting
Moving into the Ha phase, businesses begin to experiment with and adapt to these foundational practices. This is where innovation starts to take shape, as organizations explore tailored solutions, unique applications of AI, and innovative data strategies that align with their specific needs and goals.
Ri: Leading with Innovation
In the Ri stage, businesses transcend traditional applications, becoming leaders and innovators in their fields. Here, a mastery of data science and AI is leveraged to drive transformative change, deliver unprecedented value, and redefine industry standards.
Check out my other post on Reigniting Growth, How a Fortune 500 Company Transformed with Data and AI.
Conclusion: Embracing Surrender for Innovation and Success
Embracing surrender in the context of AI and data science means being open to change, continuously learning, and upholding ethical standards. This mindset is key to innovation and success in these rapidly evolving fields.
By adopting a mindset of surrender, AI and data science professionals can navigate the complexities of their fields more effectively. This approach fosters flexibility, encourages ethical practices, and drives meaningful advancements.
I encourage practitioners and enthusiasts in AI and data science to embrace this concept of surrender. By being open to change, adaptable to new ideas, and committed to ethical practices, you can contribute significantly to the growth and positive impact of these transformative fields.
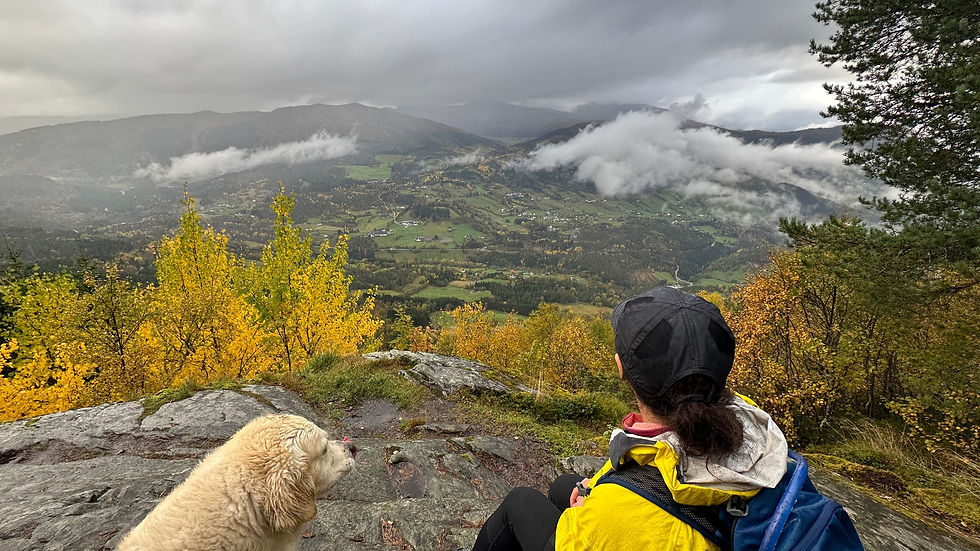